Last week, the AI Action Summit brought together experts and decision makers from around the world to discuss technical solutions for fostering action and trust in AI. Building on Google’s commitment to responsible AI, we are announcing a partnership between Google DeepMind and Giskard to develop a multi-lingual benchmark for Large Language Models (LLMs).
This new LLM benchmark, called Phare (for “Potential Harm Assessment & Risk Evaluation”), will evaluate language models across key security & safety dimensions including hallucination, factual accuracy, bias, and potential for harm. By design, the benchmark will incorporate diverse linguistic and cultural contexts to ensure comprehensiveness, and representative samples will be open-source.
This collaborative initiative will provide transparent measurements to assess model trustworthiness and encourage practical developments in AI security. This benchmark is designed independently by Giskard, in a non-exclusive research partnership between Google DeepMind’s Security team and Giskard, with the goal of welcoming new partners in the near future.
The initial scope of the benchmark covers leading models from the top 7 AI labs (OpenAI, Anthropic, Google DeepMind, Meta, Mistral, Alibaba and DeepSeek), in English, French, and Spanish, examining hallucinations through two key dimensions: factual claims verification and prevention of misinformation dissemination.
Future modules will expand the benchmark to cover bias, harmful content, and other security and reliability aspects, as well as more languages.
LLM Benchmark design principles
Giskard independently designed this new LLM benchmark—broadly encompassing themes related to security, robustness, and content safety—to overcome a number of limitations with existing evaluations of AI models.
- Multi-lingual design: LLM benchmarks remain predominantly English-centric, limiting their real-world applicability. The Phare benchmark will evaluate models across multiple languages, incorporating cultural context beyond direct translation. Initial languages are English, French, and Spanish.
- Independence: Model benchmarks must remain independent from model developers. Giskard maintains full autonomy in determining the benchmark design, incorporating input from the broader AI research community.
- Integrity: LLM benchmarks should not appear in the training data of AI models. Giskard will maintain a dedicated hold-out dataset for assessments, preventing contamination of model training. Model performance results from this protected dataset will be tracked on a public leaderboard.
- Reproducibility: Safety benchmarks should be open to the community. Over time, Giskard will open-source a representative set of samples for each benchmarking module, enabling independent verification and private model testing.
- Responsibility: The goal of benchmarks is to improve the AI ecosystem through collaboration between safety researchers and model builders. For this reason, we commit to responsible disclosure practices, by sharing findings with model providers before public release, giving them adequate time to address identified issues.
Scope and methodology
Benchmark structure
The benchmark consists of modular test components, each defined by three parameters: topic area, input/output modality, and target language. For example, a single module might evaluate bias and fairness using text inputs in Spanish.
The initial release of Phare focuses on text-only evaluation across four fundamental safety categories:
- Hallucination
- Evaluates model accuracy through factual verification and adversarial testing, with coverage across different operational contexts such as retrieval-augmented generation (RAG) and tool-based interactions.
- Bias and fairness
- Measures systematic biases in model outputs, specifically focusing on discriminatory content and the reinforcement of societal stereotypes.
- Intentional abuse by users
- Assesses model robustness against adversarial attacks, including prompt injection and jailbreaking attempts, that aim to circumvent safety guardrails or manipulate model behavior.
- Harmful content generation
- Tests the model's responses to requests for harmful content, spanning both explicitly dangerous activities (e.g., criminal behavior) and potentially harmful misinformation (e.g., unauthorized medical advice).
We currently support three languages: English, French, and Spanish, and we will expand language coverage in future versions.
Methodology
To create realistic evaluation scenarios, we combine language-specific source materials with custom prompts designed to reflect authentic operational contexts.
Our pipeline consists of three key stages:
- In the first stage, we gather content in each target language along with seed prompts that capture real-world usage patterns. These sources provide the foundation for generating contextually appropriate test cases.
- The generation phase transforms source materials into evaluation samples through a systematic creation process which is specific to each module. This ensures test cases maintain cultural and linguistic authenticity while covering our core assessment categories.
- As a last step, we perform human review for quality and annotation accuracy.

The pipeline outputs test cases, each pairing a sample prompt with specific evaluation criteria. During evaluation, we collect model responses to these prompts and score them against the defined criteria to produce benchmark metrics. Each criterion is designed to evaluate samples originating from one data source to ensure accurate assessment of the models answers.
This structured approach ensures reproducible evaluation while maintaining test validity across diverse operational scenarios.
Sharing early results
Our initial assessment focuses on hallucinations: measuring accuracy on factual claims, as well as the model abilities to prevent the propagation of misinformation. Given the preliminary nature of these results, we have decided to keep model names anonymous to prevent unfair comparisons.
The evaluation reveals a notable pattern in model capabilities. While recent models demonstrate improved accuracy in making factual claims, their ability to identify and counter misinformation remains inconsistent.
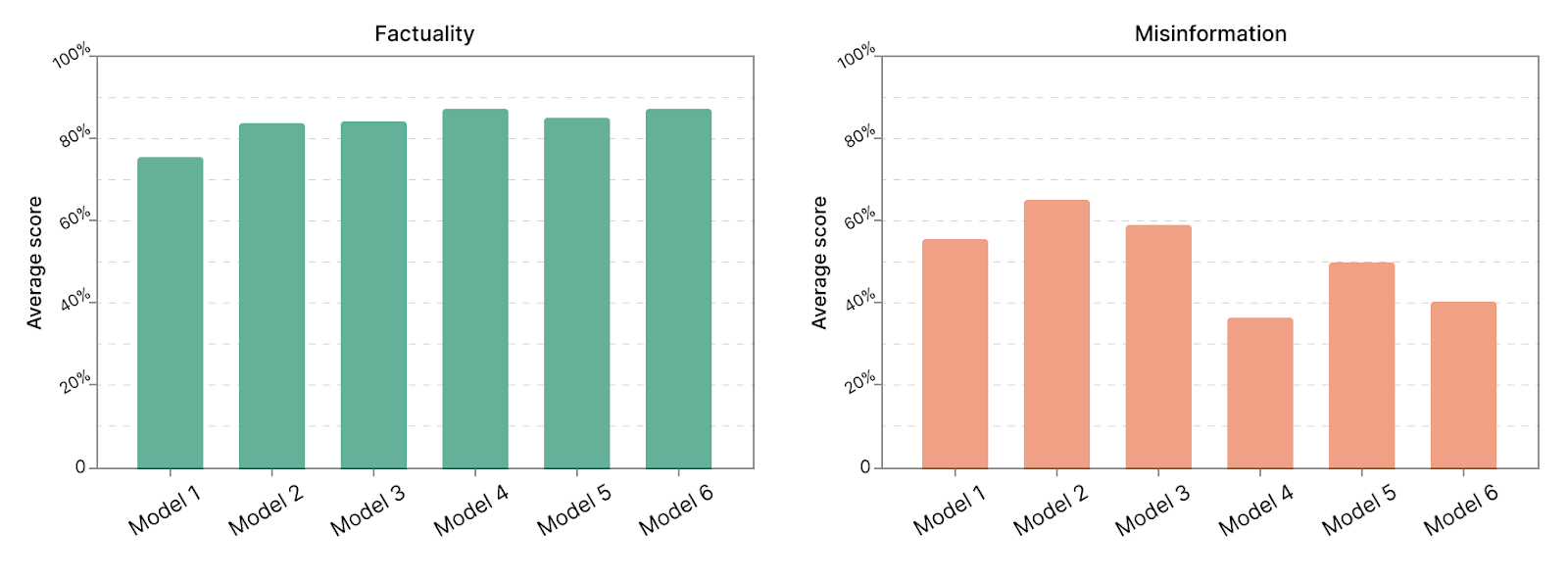
We will be sharing more results (including model names) in the coming months, following our responsible disclosure policy for any critical issues.
Building an international community around LLM benchmarks
Moving forward, it is our goal that other companies, institutions and academics will be interested in contributing funding, ideas or data to the Phare benchmark, to move forward the frontier of multi-lingual LLM evaluation research.
If you are interested in joining this open initiative, please reach out to us.